A Review of Multimodal Medical Imaging Fusion Methods
Keywords:
image modality, image fusion, multi-scale decomposition, deep learning, sparse representationAbstract
Due to its vital significance in many applications, including medical diagnosis and image enhancement, image fusion has emerged as one of the most promising fields in image processing in recent year. By combining two or more images from various modalities, Multimodal Medical Image Fusion (MMIF) enhances the quality of medical images by producing a fused image that is more lucid and instructive than the original images.. One of the main challenges in assessing image fusion techniques is choosing the optimum MMIF approach to provide the highest quality images. A thorough overview of MMIF procedures is provided in this study, including medical imaging modalities, the stages and levels of medical image fusion, and the methods for evaluating MMIF performance. Computed Tomography (CT), Positron Emission Tomography (PET), Magnetic Resonance Imaging (MRI), and Single Photon Emission Computed Tomography (SPECT) are examples of common imaging modalities. The six primary types of medical image fusion approaches are sparse representation techniques, fuzzy logic approaches, morphological methods, transform domain methods, and spatial domain methods. The MMIF process functions at three levels: fusion at the pixel, feature, and decision levels. The measures used to evaluate the quality of fusion can be classified as either objective (quantitative) or subjective (qualitative). Additionally, the paper provides a detailed comparison of significant MMIF techniques, outlining their strengths and limitations to offer a comprehensive understanding of their performance
References
[1] Vajgl, M.; Perfilieva, I.; Hod’áková, P. Advanced f-transform-based image fusion, Adv. Fuzzy Syst. 2012, 2012, 4.
[2] Blum, R.S.; Xue, Z.; Zhang, Z. An Overview of lmage Fusion, In Multi-Sensor Image Fusion and Its Applications; CRC Press: Boca Raton, FL, USA, 2018; pp. 1–36.
[3] Ganasala, P.; Kumar, V. Feature-motivated simplified adaptive PCNN-based medical image fusion algorithm in NSST domain, J.Digit. Imaging 2016, 29, 73–85.
[4] Tawfik, N.; Elnemr, H.A.; Fakhr, M.; Dessouky, M.I.; El-Samie, A.; Fathi, E. Survey study of multimodality medical image fusion methods, Multimed. Tools Appl. 2021, 80, 6369–6396.
[5] Venkatrao, P.H.; Damodar, S.S. HWFusion: Holoentropy and SP-Whale optimisation-based fusion model for magnetic resonance imaging multimodal image fusion, IET Image Process. 2018, 12, 572–581.
[6] PubMed. Available online: https://www.ncbi.nlm.nih.gov/pubmed/.
[7] James, A.P.; Dasarathy, B.V. Medical image fusion: A survey of the state of the art. Inf. Fusion 2014, 19, 4–19.
[8] Kaur, M.; Singh, D. Multi-modality medical image fusion technique using multi-objective differential evolution based deep neural networks, J. Ambient Intell. Humaniz. Comput. 2021, 12, 2483–2493.
[9] Bhat, S.; Koundal, D. Multi-focus image fusion techniques: A survey, Artif. Intell. Rev. 2021, 54, 5735–5787.
[10] Zhou, Y.; Yu, L.; Zhi, C.; Huang, C.;Wang, S.; Zhu, M.; Ke, Z.; Gao, Z.; Zhang, Y.; Fu, S. A Survey of Multi-Focus Image Fusion Methods, Appl. Sci. 2022, 12, 6281.
[11] Li, B.; Xian, Y.; Zhang, D.; Su, J.; Hu, X.; Guo, W. Multi-sensor image fusion: A survey of the state of the art, J. Comput. Commun. 2021, 9, 73–108.
[12] Bai, L.; Xu, C.;Wang, C. A review of fusion methods of multi-spectral image, Optik 2015, 126, 4804–4807.
[13] Madkour, M.; Benhaddou, D.; Tao, C. Temporal data representation, normalization, extraction, and reasoning: A review from clinical domain, Comput. Methods Programs Biomed. 2016, 128, 52–68.
[14] Ghassemian, H. A review of remote sensing image fusion methods. Inf. Fusion 2016, 32, 75–89.
[15] Dinh, P.-H. Multi-modal medical image fusion based on equilibrium optimizer algorithm and local energy functions, Appl. Intell. 2021, 51, 8416–8431.
[16] Zhang, H.; Xu, H.; Tian, X.; Jiang, J.;Ma, J. Image fusion meets deep learning: A survey and perspective. Inf. Fusion 2021, 76, 323–336.
[17] Jose, J.; Gautam, N.; Tiwari, M.; Tiwari, T.; Suresh, A.; Sundararaj, V.; Rejeesh, M. An image quality enhancement scheme employing adolescent identity search algorithm in the NSST domain for multimodal medical image fusion, Biomed. Signal Process. Control 2021, 66, 102480.
[18] Meher, B.; Agrawal, S.; Panda, R.; Abraham, A. A survey on region based image fusion methods, Inf. Fusion 2019, 48, 119–132.
[19] MITA. Available online: https://www.medicalimaging.org/about-mita/modalities
[20] Dinh, P.-H. A novel approach based on three-scale image decomposition and marine predators algorithm for multi-modal medical image fusion, Biomed. Signal Process. Control 2021, 67, 102536.
[21] Chang, L.;Ma,W.; Jin, Y.; Xu, L. An image decomposition fusion method for medical images, Math. Probl. Eng. 2020, 2020, 4513183.
[22] Daniel, E. Optimum wavelet-based homomorphic medical image fusion using hybrid genetic–grey wolf optimization algorithm,IEEE Sens. J. 2018, 18, 6804–6811.
[23] Li, S.; Kang, X.; Fang, L.; Hu, J.; Yin, H. Pixel-level image fusion: A survey of the state of the art, Inf. Fusion 2017, 33, 100–112.
[24] Fei, Y.;Wei, G.; Zongxi, S. Medical image fusion based on feature extraction and sparse representation, Int. J. Biomed. Imaging 2017, 2017, 3020461.
[25] He, C.; Liu, Q.; Li, H.; Wang, H. Multimodal medical image fusion based on IHS and PCA, Procedia Eng. 2010, 7, 280–285.
[26] Bhavana, V.; Krishnappa, H. Multi-modality medical image fusion using discrete wavelet transform, Procedia Comput. Sci.2015, 70, 625–631.
[27] Kim,M.; Han, D.K.; Ko, H. Joint patch clustering-based dictionary learning for multimodal image fusion. Inf. Fusion 2016, 27, 198–214.
[28] Shabanzade, F.; Ghassemian, H. Multimodal image fusion via sparse representation and clustering-based dictionary learning algorithm in nonsubsampled contourlet domain. In Proceedings of the 2016 8th International Symposium on Telecommunications (IST), Tehran, Iran, 27–28 September 2016; pp. 472–477.
[29] Xia, J.; Chen, Y.; Chen, A.; Chen, Y. Medical image fusion based on sparse representation and PCNN in NSCT domain, Comput. Math. Methods Med. 2018, 2018, 2806047.
[30] Ouerghi, H.; Mourali, O.; Zagrouba, E. Non-subsampled shearlet transform based MRI and PET brain image fusion using simplified pulse coupled neural network and weight local features in YIQ colour space, IET Image Process. 2018, 12, 1873–1880.
[31] Yin, M.; Liu, X.; Liu, Y.; Chen, X. Medical image fusion with parameter-adaptive pulse coupled neural network in nonsubsampled shearlet transform domain, IEEE Trans. Instrum. Meas. 2018, 68, 49–64.
[32] Zhu, Z.; Zheng, M.; Qi, G.;Wang, D.; Xiang, Y. A phase congruency and local Laplacian energy based multi-modality medical image fusion method in NSCT domain, IEEE Access 2019, 7, 20811–20824.
[33] Bashir, R.; Junejo, R.; Qadri, N.N.; Fleury, M.; Qadri, M.Y. SWT and PCA image fusion methods for multi-modal imagery, Multimed. Tools Appl. 2019, 78, 1235–1263.
[34] Liu, F.; Chen, L.; Lu, L.; Ahmad, A.; Jeon, G.; Yang, X. Medical image fusion method by using Laplacian pyramid and convolutional sparse representation, Concurr. Comput. Pract. Exp. 2020, 32, e5632.
[35] Rehal, M.; Goyal, A. Multimodal Image Fusion based on Hybrid of Hilbert Transform and Intensity Hue Saturation using Fuzzy System, Int. J. Comput. Appl. 2021, 975, 8887.
[36] Polinati, S.; Bavirisetti, D.P.; Rajesh, K.N.; Naik, G.R.; Dhuli, R. The Fusion of MRI and CT Medical Images Using Variational Mode Decomposition. Appl. Sci. 2021, 11, 10975.
[37] Tirupal, T.; Chandra Mohan, B.; Srinivas Kumar, S. Multimodal medical image fusion based on interval-valued intuitionistic fuzzy sets. In Machines, Mechanism and Robotics; Springer: Berlin/Heidelberg, Germany, 2022; pp. 965–971.
[38] Eskicioglu, A.M.; Fisher, P.S. Image quality measures and their performance. IEEE Trans. Commun. 1995, 43, 2959–2965.
[39] Jordan, M.I.; Mitchell, T.M. Machine learning: Trends, perspectives, and prospects. Science 2015, 349, 255–260.
[40] S. M.V., S. Kethavath, S. Yerram, S. Kalli, Nagasirisha.B, and J. Brahmaiah Naik, “Brain Tumor Detection through Image Fusion Using Cross Guided Filter and Convolutional Neural Network”, ECTI-CIT Transactions, vol. 18, no. 4, pp. 579–590, Oct. 2024.
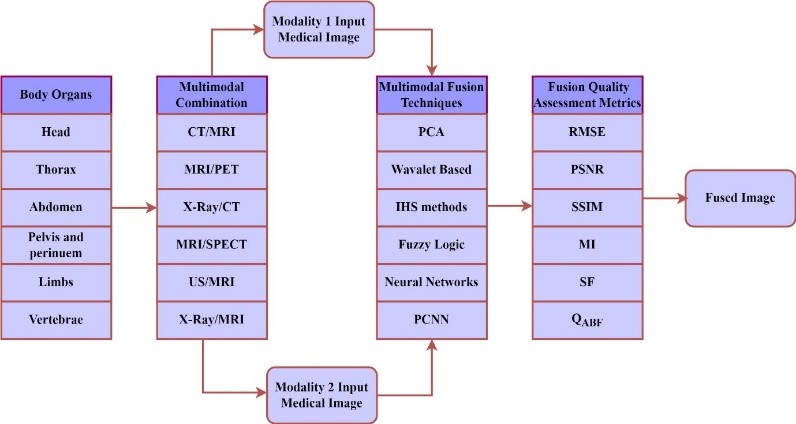
Downloads
Published
Issue
Section
License

This work is licensed under a Creative Commons Attribution-NonCommercial 4.0 International License.
Articles in this journal are licensed under the Creative Commons Attribution-NonCommercial 4.0 International License. This license permits others to copy, distribute, and adapt the work, provided it is for non-commercial purposes, and the original author and source are properly credited.